Unlocking the Power of Big Data to Transform the Lives of People with Rare Disorders
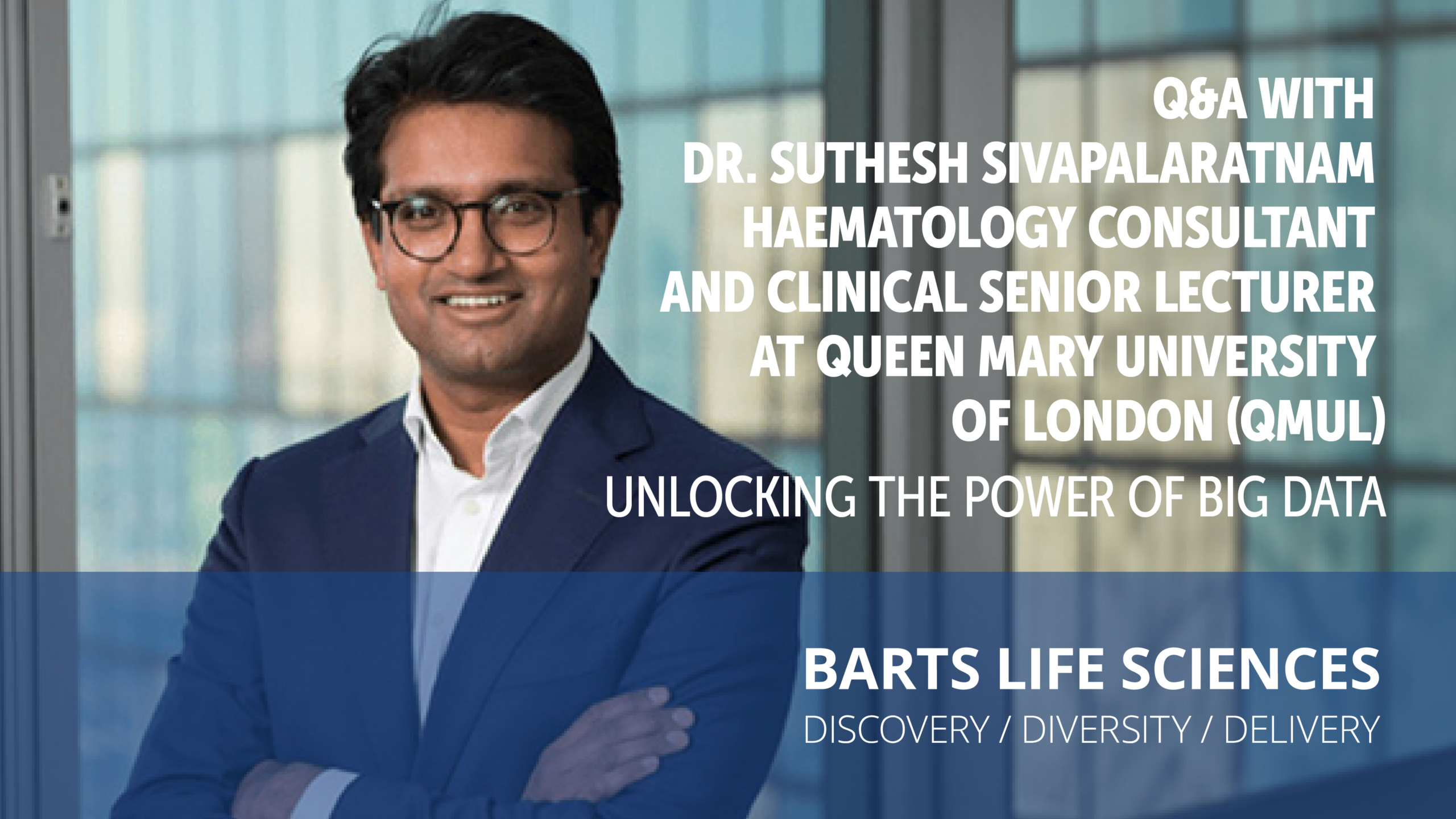
Rare diseases can feel like a puzzle—complex, often elusive, and challenging to diagnose. For many patients, the journey to understanding their condition can be long and frustrating, given that these disorders affect relatively small populations. Yet, the emergence of big data is changing the game, offering us new and exciting ways to revolutionise how we identify and treat these conditions.
In this thought leadership piece, we hear from Dr. Suthesh Sivapalaratnam, Haematology Consultant at Barts Health NHS Trust and Clinical Senior Lecturer at Queen Mary University of London (QMUL). He shares his passion for the transformative potential of big data in rare disease research. Dr. Sivapalaratnam offers valuable insights into the latest trends, the capabilities of platforms like the Barts Health Data Platform, and how collaboration and cutting-edge technology can help us tackle the unique challenges posed by rare disorders.
Through engaging real-world examples and a wealth of experience, this article reveals how artificial intelligence, machine learning, and large datasets are not just buzzwords but essential tools in accelerating diagnoses and improving patient outcomes. We’ll explore how breaking down barriers between institutions can lead to more effective care for those who need it most.
Note the views expressed herein are those solely of the author.
What trends have you seen in the use of large datasets to transform how we identify and treat patients with rare disorders?
The key trends involve a willingness to analyse data on a large scale, combined with the empowerment that comes from having the right platforms. By platforms, I mean access to patient data from the NHS. The pandemic really opened this area up, as we realised that tackling large-scale epidemics required accessing data without barriers.
Another trend is the development of enabling software and platforms. Forty years ago, this would not have been possible. We didn’t have electronic health records, the server capacity to store that data, or the analytical knowledge and compute power to process it sensibly.
Even now, when we speak about large datasets, genomics is a great example of large data. There are platforms that allow for tremendous possibilities. Rather than looking at entire sequences that would yield terabytes of data, we now have found ways to compress the data into manageable segments for analysis. In the genomics world, collaboration between institutions and methods for extracting high-level data have made this possible.
What’s most important right now is that the Government is also keen to ensure this data is used safely because there are immense benefits to doing so.
In what ways can large datasets improve the early diagnosis of rare diseases, and how does this impact patient outcomes? Ideally, what datasets are required?
Large datasets can significantly enhance the early diagnosis of rare diseases by providing comprehensive insights that individual healthcare providers may overlook due to the increasing subspecialisation and fragmentation of care. Traditionally, a general practitioner would oversee a patient’s health, piecing together clues from various interactions. However, as the healthcare system evolves, this holistic approach is becoming less common, and doctors often lack the time to synthesise all relevant information. A tool that can aggregate and analyse data helps identify patterns and potential oversights, ultimately improving patient outcomes.
With the rising number of rare disorders, it is impossible for any single practitioner to be familiar with every condition. Thus, having a system that can pinpoint specific features of rare diseases is invaluable. For instance, Barts Life Sciences has developed a tool that identifies individuals with Vaccine-Induced Immune Thrombotic Thrombocytopenia upon their arrival at the hospital by integrating primary, secondary, and vaccination data. We are also working on identifying patients with Gaucher’s disease earlier by combining all relevant data points.
Moreover, large datasets allow us to explore not only known data points but also the unexpected ways in which rare diseases can present. Doctors are often trained to diagnose based on specific symptoms, but patients may not fit the typical presentation. For example, in my clinic, I typically focused only on questions directly related to the disorder. However, upon analysing data from a phase 1 and phase 2 trial without bias, we discovered that several patients with platelet disorders reported joint bleeds, contrary to what we had been taught. This valuable data could have been lost without the robust analysis enabled by large datasets.
Ideally, what datasets are required? To maximise the effectiveness of these tools, it is essential to have high-quality, curated data on each individual. This includes comprehensive health records, genetic information, treatment histories, and any other relevant health indicators that can be systematically analysed. Having access to such rich datasets will significantly enhance our ability to identify and diagnose rare diseases early, ultimately improving patient outcomes.
What unique challenges do rare diseases pose, and what role could AI and machine learning play?
One of the unique challenges posed by rare diseases is the delay in diagnosis. Often, neither the patient nor the clinician initially considers a rare disorder, leading to a slow diagnosis. Symptoms tend to start mildly and gradually worsen over time, unlike acute conditions like a car accident, where the injury and treatment are immediate and obvious. With rare diseases, symptoms accumulate slowly, and someone needs to recognise the pattern.
This delay occurs not only because the doctor may not consider the rare disease, but also because getting the patient to the right tests and specialist centre can be a challenge. AI and machine learning could play a crucial role in helping to identify these patterns early, flagging potential rare conditions and ensuring patients receive the appropriate tests and referrals in a timelier manner.
How is the Barts Health Data Platform being used to identify patients with rare diseases? Is it purely for initial diagnosis, or does it also monitor disease progression?
The Barts Health Data Platform enhances early diagnosis through advanced algorithms tailored to our local patient population. By adapting existing models, we can identify rare diseases more swiftly.
Once patients are identified, we ensure timely referrals to appropriate services, preparing the care team in advance. Our approach extends beyond diagnosis; we prioritise effective communication with patients awaiting results, using sensitive language and providing essential support to ease their experience.
Currently, we haven’t implemented monitoring for disease progression, but we envision future capabilities for predicting progression. This would enable us to manage follow-up appointments and determine when patients can be discharged.
How important is collaboration and data sharing between institutions in improving research on rare disorders?
Collaboration and data sharing are absolutely crucial. Each institution has its own area of expertise, and by combining this knowledge and breaking down barriers, we can significantly improve patient care. If patients visit different centres, we should embrace that collaboration. It’s important to work not only with other NHS institutions but also with companies and the pharmaceutical industry. This is not the time to divide people into “good” or “bad” categories—we all share the same goal, which is improving outcomes for patients. The key is finding the best way to achieve that together. One such example of collaboration is project Sirius, a project we are running collaboratively with Sanofi UK.
In the past data sharing between primary and secondary healthcare made it possible to detect people at risk of having Vaccine Induced Thrombocytopenia and Thrombosis.
What are the main barriers preventing clinicians from participating in the big data revolution?
There are several significant issues. Firstly, many clinicians lack the time necessary to engage in research due to their demanding schedules filled with patient care responsibilities. This time constraint makes it challenging for them to delve into the complexities of big data. Secondly, some clinicians may feel intimidated by the computational aspects of data analysis or lack familiarity with the regulatory landscape surrounding data use. This uncertainty can lead to hesitation or reluctance to explore the field. Additionally, there might be concerns about the relevance of big data to their specific practice areas, which can further hinder engagement. Creating a supportive environment that addresses these barriers is essential for fostering clinician participation in the big data revolution.
How can we make the Barts Health Data Platform more accessible for clinicians?
We should enhance accessibility by pairing interested clinicians with data scientists, similar to the collaboration I’ve had with Sophie Williams and Concetta Piazzese from Barts Life Sciences, fostering mentorship and knowledge exchange. Additionally, we should have an intuitive, easy-to-use portal where clinicians can pose simple research questions, ensuring the interface is designed to be user-friendly and almost “dummy-proof.” This would help bridge the gap between clinical expertise and data analysis. Furthermore, given the challenges clinicians face when navigating IT processes, having a dedicated case manager could be invaluable. This resource would guide clinicians through the technical hurdles, reducing frustration and increasing the likelihood of successful engagement with big data initiatives. Such measures would not only make the Platform more accessible but also empower clinicians to harness the potential of big data in their practice.
While big data offers significant potential, what are its limitations in the context of rare diseases? Are there scenarios where reliance on big data could lead to misleading conclusions or unintended consequences?
The main concern with big data is ensuring the quality of the data itself. Having vast amounts of data doesn’t always mean it’s useful or reliable. It’s crucial to assess the depth of the data and understand how it was acquired. Correct interpretation is also key—one example is using Natural Language Processing (NLP), where the placement of a word like “no” can completely change the meaning, such as whether a patient has an enlarged spleen or “no” enlarged spleen.
We must ensure these tools work correctly and are applied appropriately. Another critical factor is data security. Any tool we use must guarantee that patients’ data remains secure, minimising the risk of data breaches and ensuring no harm comes to the patient. That’s why the tools, methods, and collaborations we use must adhere to the highest ethical and governance standards, so patients always feel safe coming to hospital and sharing their concerns.
AI and machine learning models can sometimes reflect or even amplify biases. How do you ensure that methods for identifying patients with rare diseases or predicting outcomes are free from such biases?
You’re absolutely right. One way to minimise bias is by ensuring we sample a diverse range of populations. To draw an analogy from genomics, which is my background, if you only study a mutation common in a white Caucasian population, that same mutation might present very differently in a Bengali population. When diagnosing rare diseases, we often focus on mutations that are considered rare. However, a mutation that is rare in the Caucasian population could be quite common in the Bengali population. If we don’t account for that, we might reach incorrect conclusions when diagnosing someone from the Bengali community. By including diverse populations in research, we can help prevent these kinds of errors and ensure our methods are more accurate and inclusive.
Given that rare diseases affect a relatively small population, how do you justify the allocation of resources for big data projects in this field compared to more common diseases? Is there a risk of these efforts not being cost-effective?
I think you’re right that rare disorders can serve as models for other conditions. We begin by identifying people with rare disorders through algorithms in their electronic health records, but these same tools can also be used to identify other rare conditions and even high-risk individuals with common disorders, making them eligible for studies and trials.
The burden of rare disorders on healthcare tends to be high—both the diagnostics and the treatments are expensive because these conditions are so rare. One way to improve this is by diagnosing people earlier, potentially reducing the need for costly treatments. Ultimately, we need to work on both ends of the spectrum—common and rare disorders—to ensure better outcomes and cost-effectiveness.
Finally, based on your experience, what do you find most exciting about using large data and the Barts Health Data Platform to tackle the challenges of rare diseases?
What excites me most is how far Barts Health has come. They’ve developed an incredible Platform—a resource that many would love to have—built with the support of Barts Charity and the technological expertise of people like Sarah Jensen, Charles Gutteridge, Steven Newhouse and Sophie Williams. They’ve turned it into a tool that people can and will want to use, which is truly remarkable.
It’s also exciting to see a more diverse group of participants engage in research. What’s even more amazing is that it’s not just a Data Platform, but a hub of expertise for handling and analysing the data, and for developing and deploying algorithms.
While many start-up companies might have the financial backing, they often lack experience with real patients, the complexity of healthcare, or the underlying data structures. At Barts, we can handle the real, hands-on work of accessing and interpreting the data, which is what makes this so exciting.
About the author
Dr. Suthesh Sivapalaratnam is a leading figure at one of the largest haemophilia practices globally, based at the comprehensive care centre at Barts Health NHS Trust, where the MDT team manages a patient cohort of 2,350 individuals with rare inherited bleeding disorders. His training began in Amsterdam under the esteemed Professor Levi and Prof Ouwehand (University of Cambridge), where he obtained his PhD in Molecular Basis of Cardiovascular Disease.
Following his PhD, Dr. Sivapalaratnam conducted post-doctoral research at Massachusetts General Hospital and The Broad Institute in Boston, where he deepened his expertise in genomics under the mentorship of Professor Sekar Kathiresan. He currently leads the paediatric haemostasis and thrombosis service, serves as the genomics lead and multidisciplinary team (MDT) chair for North London, and is the academic lead for clinical haematology.
As an academic theme lead in precision medicine, Dr. Sivapalaratnam focuses on genomics and data sciences, acting as a principal investigator on innovative clinical trials. His work with Barts Life Sciences is dedicated to the early detection of rare disorders and leveraging big data to enhance health outcomes. With over 70 peer-reviewed publications and a Google Scholar h-index of 30, he is a prominent voice in the field. Additionally, he actively participates in various committees, including chairing the genomics working party of the UK Haemophilia Centre Doctors’ Organisation (UKHCDO) and the International Society on Thrombosis and Haemostasis (ISTH). He also contributes advisory expertise to the Haemophilia Society and various biotech initiatives.